"We want AI as a helpful assistant, not as an independent being"
Benjamin Grewe is Professor of Neuroinformatics and Neural Systems at the Institute of Neuroinformatics (INI) of ETH Zurich and the University of Zurich and a researcher at the ETH AI Center. In the interview, he talks about his fascination for biologically inspired algorithms and the advantages of the newly developed language model SwissGPT over ChatGPT.
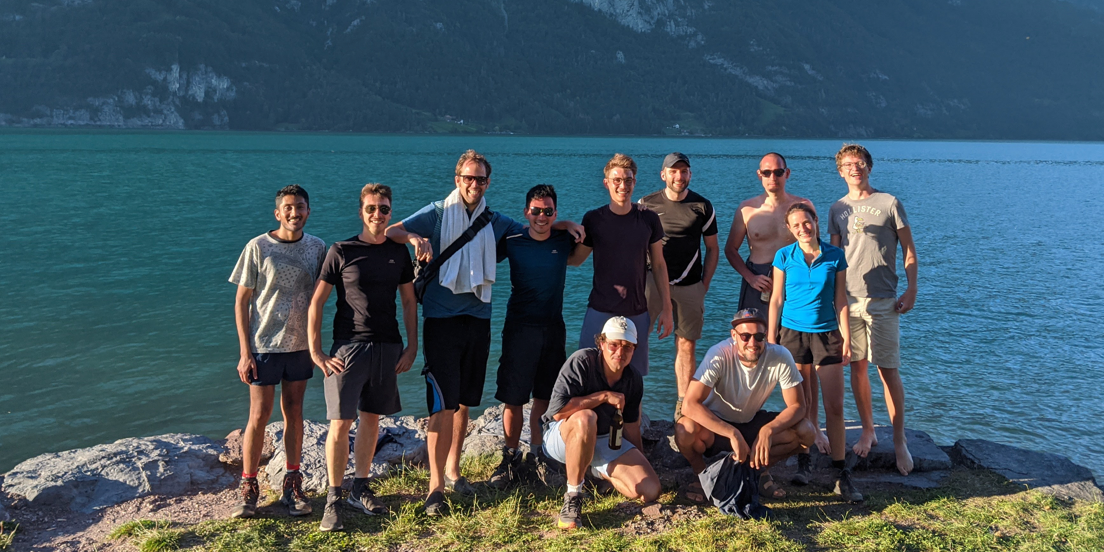
What is your field of research?
My research area covers two main topics. Firstly, we study the functional principle of the brain and its similarity to a computer. We analyse how the brain processes information to produce behaviour and language, and how these abilities emerge. The goal of my research is to understand why we have these abilities to control our behaviour in different situations so that we achieve our goals.
The other direction of my research concerns engineering, Machine Learning and theoretical approaches such as Artificial Neural Network learning. We are evaluating the state of machine learning in neural networks, especially ChatGPT. Our goal is to understand why certain tasks can be mastered while the algorithm fails at others. We want to make these algorithms more biologically inspired, similar to the human learning process. In the long run, I aim to develop an algorithm that solves tasks the way I would, by understanding my approach and understanding my goals.
You are a trained physicist. Could you explain how you came to neuroinformatics and artificial intelligence?
I was initially an enthusiastic physicist and deeply rooted in particle physics. Then, during my studies in Heidelberg, my enthusiasm for neuroscience was awakened in a biophysics lecture. In this lecture, we observed live how neurons process information and change in the brain. So I switched to biophysics, specialised in brain research and wrote my thesis. This brought me into the exciting field of neuroscience.
I did my PhD in neuroinformatics at ETH Zurich, where I developed a microscope to precisely image fast neuronal activity. Neurons react in milliseconds, so we needed fast and high-resolution measurement methods.
Have you managed to better understand the human brain in the meantime?
Yes, indeed! However, this has raised more questions than before. But at its core, it is positive to understand more and at the same time to seek further insights. We have already made some significant steps in this process. We have seen how the coding of a stimulus changes when it takes on behavioural meaning. A concrete example: a stimulus that triggers fear is not only recognised, but also interpreted as a kind of "warning".
We also found out how this stimulus is then translated into behaviour in the next step. In the hierarchically structured brain, where information is processed step by step, the "warning" for the stimulus appears in the anterior, prefrontal cortex. It signals heightened attention and allows us to predict which action will be selected as early as three to four seconds before an action is performed. All of this was based solely on the sensory stimulus which is transformed in the brain into a learned coding of behavioural plans.
But surely there is also innate, instinctive behaviour that is not based on experience?
There are some stimuli, especially in relation to fears, where it is not clear whether they are innate or not. It seems that they are very basic behaviours and that they are already preset in our brain. Of course, we would have to explicitly teach a robot everything, such as running away from a lion, because it does not have this innate behaviour by nature.
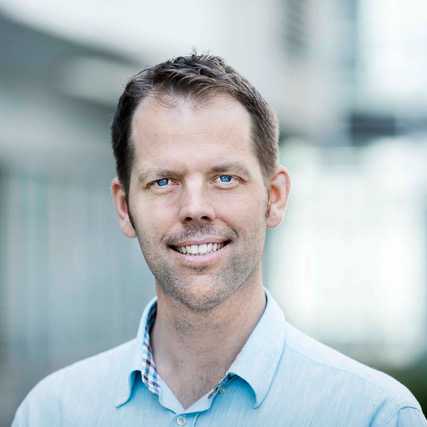
“We would have to explicitly teach a robot to run away from a lion because it does not have this innate behaviour by nature.”Prof. Benjamin Grewe![]()
What impact does your research have on society?
What we are researching is very different from what we currently see in AI, such as ChatGPT. ChatGPT has been trained to respond to input such as images or text and then predict the next word. But it can't effectively generate behaviour or control physical interaction.
Our research, on the other hand, is concerned with understanding and developing biological and robotic systems that can not only take in abstract visual impressions, but also comprehend what action plans or behavioural options they have depending on the situation. One of our projects at the ETH AI Center, for example, is concerned with developing a "Robotic Action Transformer". Here, it is fascinating that the robot can understand almost everything that is communicated to it via language and then carries out corresponding actions. This results in exciting areas of application in the industrial or medical sector, such as a surgical robot that we are currently training and developing virtually.
What important contributions can electrical engineering make to this?
The algorithms we develop to control learning processes have to run on hardware, currently on GPUs. We have recently made great progress here and I believe that in a few years we will be able to develop our own algorithms for so-called neuromorphic processors that consume significantly less energy. Electrical engineering provides us with important technical foundations for this.
With the start-up AlpineAI, you are developing a Swiss alternative to ChatGPT. What can this language model do?
With SwissGPT, we are pursuing the goal of automating stereotypical computer work. When we interact with ChatGPT today, our input goes to a server abroad where our data is stored. Caution, regulation and trust are important here. In this respect, we are well positioned in Switzerland. We implement our SwissGPT products directly in the companies, for example on the company's internal servers, so that the data stays there.
What dangers do you see with regard to artificial intelligence? And how human will AIs be in the future?
The biggest danger I see at the moment is not that algorithms do undesirable things, but rather that people with different interests could use the technologies. This raises the question of how we can intervene in time to ensure the right direction.
I believe that in the future we will have AIs that are human-like. However, this also raises the question of how far we actually want this to go. For example, I don't want to sit in a car that then tells me it doesn't feel like driving today. We need to think about how we can give these algorithms meaningful goals. However, we may not want to give them the same goals as a human. Nevertheless, we want these systems to understand us, but on a level of a helpful assistant and not on the level of an independent being.
How is your research group structured?
Half of my group consists of brain researchers and half of experts in computational neuroscience and machine learning who want to develop bioplausible systems similar to the brain. The collaboration between the two subgroups is enormously inspiring. The proportion of women is a pleasing 30 to 40 percent, and we have very international members, e.g. from Germany, the Netherlands, Belgium, America and Morocco.
What lectures are you giving this semester?
The lecture I developed at Master's level, which I am constantly adapting to the latest developments in artificial intelligence, is entitled "Learning in Deep Artificial and Biological Neural Networks". This lecture is about parallels between biological intelligence and AI, current AI challenges, discrepancies and the possibility of transferring ideas between the fields to solve questions in other disciplines and generate new hypotheses.
We start with biological principles and then delve into machine learning, which is quite a challenge for students. The exercises cover both understanding biology and programming biological networks. We create synergies here so that students from different disciplines can understand the subject matter and work together in an interdisciplinary way.
What is the biggest challenge with AI at the moment?
What is still missing is the better integration of goals into AI systems. In our human actions, we always have a goal in mind, be it short-term or abstract-long-term. These aspects are not yet included in the "Robotic Transformer".
We need to think about what data we use, how we train and how the architecture of the system is designed. What do we want the systems to do in the first place? If we reach this point, we can manage to train systems that don't quite get to the level of humans, but are still at a higher level than ChatGPT. This will probably take another five to ten years.
Editorial assistance: ChatGPT